(Let me know if you find any problems!)
The Live Turnout Tracker is back!
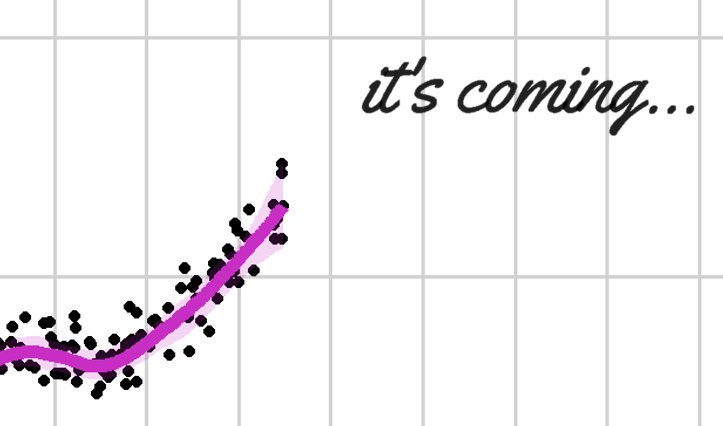
I’ve projected that Philadelphia is on pace for a record turnout year, estimating that 460,000 Philadelphians will vote on November 6th. This would beat every midterm election at least since 2002. Can we really do it? Let’s watch in real time!
What it is
The Turnout Tracker is a tool for all of us voters to share our information to generate real-time vote counts on Election Day. When you vote in Philadelphia, just record your Voter Number at your precinct (you’ll be able to see it when you sign in). Then share it here: bit.ly/sixtysixturnout
I’ll aggregate the submissions, do a little bit of math, and estimate the current number of voters across the city. You can follow it here on election day: jtannen.github.io/election_tracker.html
The Model
The model is largely the same as the primary. You can read about what it does here: announcing-the-live-election-tracker.html
I’ve changed a few things, given the Primary’s post-mortem. In May, I used too much smoothing, and the result was that I missed the collapse in voting between 7-8pm due to the ill-timed thunderstorm. Worse, so many people participated that I could have gotten away with a lot less smoothing, so the error was avoidable.
This time around, I’ve built an adaptive smoother based on the number of datapoints. In connection, I’m going to bootstrap the uncertainty for every prediction, instead of using a parametric estimate. Non-parametric smoothers (the algorithm that gives me my curvy line) are particularly noisy around the edges. Unfortunately, the edge is exactly what we care about most: the final turnout is the estimate right at 8pm, the edge of our window. Making the model more responsive to short-term changes in trajectory also means it could overfit data points right at 7:59, and really mess up the curve. Bootstrapping addresses this by resampling the observed datapoints at random, giving a range of estimates that aren’t all dependent on the same points. The result is that it should correctly identify the true amount of noise right at 8pm, and give wide enough uncertainty accordingly. This will make my estimates less certain–and the plausible range larger–but hopefully allow the model to be robust to drastic end-of-day bends. And the more people that participate, the more the model will adapt to small signals, and the better result we’ll get.
What I need from you
Vote! Get your friends to vote! Then have everyone share their voter number at bit.ly/sixtysixturnout. Y’all came up huge last time around, and I’m hoping we can do it again.
See you on November 6th!